Lately I’ve been doing a little bit of research on voting methods. In particular, I’ve been fascinated by this idea of measuring Bayesian Regret. Unfortunately, many of the supporting links on rangevoting.org are dead. With a little detective work I managed to track down the original study and the supporting source code.
Looking at this information critically, one my concerns was the potential for bias in the study. This is the only study I could find taking this approach, and the information is hosted on a site that is dedicated to the support of the method proved most effective by the study. This doesn’t necessarily mean the result is flawed, but it’s one of the “red flags” I look for with research. With that in mind, I did what any skeptic should: I attempted it replicate the results.
Rather than simply use the provided source code, I started writing my own simulation from scratch. I still have some bugs to work out before I release my code, but the experience has been very educational so far. I think I’ve learned more about these voting methods by fixing bugs in my code than reading the original study. My initial results seem consistent with Warren Smith’s study but there’s still some kinks I need to work out.
What I’d like to do in this post is go over a sample election that came up while I was debugging my program. I’m hoping to accomplish a couple things by doing so. First, I’d like to explain in plain English what exactly the simulation is doing. The original study seems to be written with mathematicians in mind and I’d like for these results to be accessible to a wider audience. Second, I’d like to outline some of the problems I ran into while implementing the simulation. It can benefit me to reflect on what I’ve done so far and perhaps some reader out there will be able to provide input on these problems that will point me in the right direction.
Pizza Night at the Election House
It’s Friday night in the Election household, and that means pizza night! This family of 5 takes a democratic approach to their pizza selection and conducts a vote on what time of pizza they should order. They all agree that they should get to vote on the pizza. The only problem is that they can’t quite agree on how to vote. For the next 3 weeks, they’ve decided to try out 3 different election systems: Plurality, Instant-Runoff, and Score Voting.
Week 1: Plurality Voting
The first week they use Plurality Voting. Everyone writes down their favorite pizza and which ever pizza gets the most votes wins.
The youngest child votes for cheese. The middle child votes for veggie. The oldest votes for pepperoni. Mom votes for veggie, while dad votes for hawaiian.
With two votes, veggie pizza is declared the winner.
Mom and the middle child are quite happy with this result. Dad and the two others aren’t too excited about it. Because the 3 of them were split on their favorites, the vote went to an option that none of them really liked. They feel hopeful that things will improve next week.
Week 2: Instant Run-off Voting
The second week they use Instant Run-off Voting. Since the last election narrowed down the pizzas to four options, every lists those four pizzas in order of preference.
The youngest doesn’t really like veggie pizza, but absolutely hates pineapple. Ranks cheese 1st, pepperoni 2nd, veggie 3rd,and hawaiian last.
The middle child is a vegetarian. Both the hawaiian and pepperoni are bad options, but at least the hawaiian has pineapple and onions left over after picking off the ham. Ranks veggie 1st, cheese 2nd, hawaiian 3rd and pepperoni last.
The oldest child moderately likes all of them, but prefers fewer veggies on the pizza. Ranks pepperoni 1st, cheese 2nd, hawaiian 3rd and veggie last.
Dad too moderately likes all of them, but prefers the options with meat and slightly prefers cheese to veggie. Ranks hawaiian 1st, pepperoni 2nd, cheese 3rd and veggie last.
Mom doesn’t like meat on the pizza as much as Dad, but doesn’t avoid it entirely like the middle child. Ranks veggie 1st, cheese 2nd, pepperoni 3rd and hawaiian last.
Adding up the first place votes gives the same result as the first election: 2 for veggie, 1 for hawaiian, 1 for pepperoni and 1 for cheese. However, under IRV the votes for the last place pizza get transferred to the next ranked pizza on the ballot.
However, there’s something of a problem here. There’s a 3-way tie for last place!
A fight nearly breaks out in the Election house. Neither dad, the older or youngest want their favorite to be eliminated. The outcome of the election hinges on whose votes get transferred where!
Eventually mom steps in and tries to calm things down. Since the oldest prefers cheese to hawaiian and the youngest prefers pepperoni to hawaiian, it makes sense that dad’s vote for hawaiian should be the one eliminated. Since the kids agree with mom’s assessment, dad decides to go along and have his vote transferred to pepperoni.
Now the score is 2 votes for veggie, 2 votes for pepperoni, and 1 vote for cheese. Since cheese is now the lowest, the youngest childs vote gets transferred to the next choice: pepperoni. With a vote of 3 votes to 2, pepperoni has a majority and is declared the winner.
The middle child is kind of upset by this result because it means she’ll need to pick all the meat off her pizza before eating. Mom’s not exactly happy with it either, but is more concerned about all the fighting. They both hope that next week’s election will go better.
Week 3: Score Voting
The third week the Election family goes with Score Voting. Each family member assigns a score from 0 to 99 for each pizza. The pizza with the highest score is declared the winner. Everyone wants to give his/her favorite the highest score and least favorite the lowest, while putting the other options somewhere in between. Here’s how they each vote:
The youngest rates cheese 99, hawaiian 0, veggie 33 and pepperoni 96.
The middle child rates cheese 89, hawaiian 12, veggie 99 and pepperoni 0.
The oldest child rates cheese 65, hawaiian 36, veggie 0 and pepperoni 99.
Dad rates cheese 13, hawaiian 99, veggie 0 and pepperoni 55.
Mom rates cheese 80, hawaiian 0, veggie 99 and pepperoni 40.
Adding all these scores up, the finally tally is 346 for cheese, 147 for hawaiian, 231 for veggie and 290 for pepperoni. Cheese is declared the winner. Some of them are more happier than others, but everyone’s pretty much okay with cheese pizza.
Comparing the Results
Three different election methods. Three different winners. How do we tell which election method is best?
This is where “Bayesian Regret” comes in.
With each of these 3 elections, we get more and more information about the voters. First week, we get their favorites. Second week, we get an order of preference. Third week, we get a magnitude of preference. What if we could bypass the voting altogether and peak instead the voter’s head to see their true preferences? For the family above, those preferences would look like this:
|
cheese |
hawaiian |
veggie |
pepperoni |
youngest |
99.92% |
2.08% |
34.25% |
95.79% |
middle |
65.95% |
10.09% |
73.94% |
0.61% |
oldest |
74.55% |
66.76% |
57.30% |
83.91% |
dad |
52.13% |
77.03% |
48.25% |
64.16% |
mom |
87.86% |
39.79% |
99.72% |
63.94% |
These values are the relative “happiness levels” of each option for each voter. It might help to visualize this with a graph.
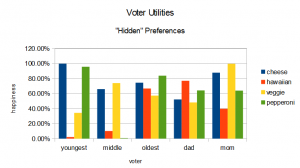
If we had this data, we could figure out which option produced the highest overall happiness. Adding up these “happiness” units, we get 380 for cheese, 195 for hawaiian, 313 for veggie and 308 for pepperoni. This means the option that produces the most family happiness is the cheese pizza. The difference between the max happiness and the outcome of the election gives us our “regret” for that election. In this case: the plurality election has a regret of 67, the IRV election has a regret of 72, and the score voting election has a regret of 0 (since it chose the best possible outcome).
Now keep in mind that this is only the regret for this particular family’s pizza selection. To make a broader statement about which election method is the best, we need to look at all possible voter preferences. This is where our computer simulation comes in. We randomly assign a number for each voter’s preference for each candidate, run the elections, calculate the regret, then repeat this process over and over to average the results together. This will give us an approximation of how much regret will be caused by choosing a particular voting system.
Open Questions
In writing my simulation from scratch, I’ve run into a number of interesting problems. These aren’t simply programming errors, but rather conceptual differences between my expectations and the implementation. Some of these questions might be answerable through more research, but some of them might not have a clear cut answer. Reader input on these topics is most welcome.
Implementing IRV is complicated
Not unreasonably hard, but much more so than I had originally anticipated. It seemed easy enough in theory: keep track of the candidates with the lowest number of votes and eliminate them one round at a time. The problem that I ran into was that in small elections, which I was using for debugging, there were frequently ties between low ranked candidates in the first round (as in the case story above). In the event of a tie, my code would eliminate the candidate with the lower index first. Since the order of the candidates was essentially random, this isn’t necessarily an unfair method of elimination. However, it did cause some ugly looking elections where an otherwise “well qualified” candidate was eliminated early by nothing more than “bad luck”.
This made me question how ties should be handled in IRV. The sample elections my program produced showed that the order of elimination could have a large impact on the outcome. In the election described above, my program actually eliminated “cheese” first. Since the outcome was the same, it didn’t really matter for this example. However, if the random ordering of candidates had placed “pepperoni” first then “cheese” would have won the election! Looking at this probabilistically, the expected regret for this example would be 1/3*0+2/3*72 = 48. A slight improvement, but the idea of non-determinism still feel out of place.
I started looking into some alternative methods of handling ties in IRV. For a simulation like this, the random tie-breaker probably doesn’t make a large difference. With larger numbers of voters, the ties get progressively more unlikely anyways. However, I do think it could be interesting to compare the Bayesian Regret among a number of IRV variations to see if some tie breaking mechanisms work better than others.
Bayesian Regret is a societal measure, not individual
When I first started putting together my simulation, I did so “blind”. I had a conceptual idea of what I was trying to measure, but was less concerned about the mathematical details. As such, my first run produced some bizarre results. I still saw a difference between the voting methods, but at a much different scale. In larger elections, the difference between voting methods was closer to factor of .001. With a little bit of digging, and double-checking the mathematical formula for Bayesian Regret, I figured out I did wrong. My initial algorithm went something like this:
I took the difference between the utility of each voter’s favorite and the candidate elected. This gave me an “unhappiness” value for each voter. I averaged the unhappiness of all the voters to find the average unhappiness caused by the election. I then repeated this over randomized elections and kept a running average of the average unhappiness caused by each voting method. For the sample election above, voters are about 11% unhappy with cheese versus 24% or 25% unhappy with veggie and pepperoni respectively.
I found this “mistake” rather intriguing. For one thing, it produced a result that kind of made sense intuitively. Voters were somewhat “unhappy” no matter which election system was used. Even more intriguing was that if I rescaled the results of an individual election, I found that they were distributed in close to the same proportions as the results I was trying to replicate. In fact, if I normalized the results from both methods, i.e. R’ = (R-MIN)/(MAX-MIN), then they’d line up exactly.
This has become something of a dilemma. Bayesian Regret measures exactly what it says it does — the difference between the best option for the society and the one chosen by a particular method. However, it produces a result that is somewhat abstract. On the other hand, my method produced something a little more tangible — “average unhappiness of individual voters” — but makes it difficult to see the differences between methods over a large number of elections. Averaging these unhappiness values over a large number of elections, the results seemed to converge around 33%.
Part of me wonders if the “normalized” regret value, which aligns between both models, might be a more appropriate measure. In this world, it’s not the absolute difference between the best candidate and the one elected but the difference relative to the worst candidate. However, that measure doesn’t really make sense in a world with the potential for write-in candidates. I plan to do some more experimenting along these lines, but I think the method of how to measure “regret” is a very an interesting question in itself.
“Honest” voting is more strategic than I thought
After correcting the aforementioned “bug”, I ran into another troubling result. I started getting values that aligned with Smith’s results for IRV and Plurality, but the “Bayesian Regret” of Score Voting was coming up as zero. Not just close to zero, but exactly zero. I started going through my code and comparing it to Smith’s methodology, when I realized what I did wrong.
In my first implementation of score voting, the voters were putting their internal utility values directly on the ballot. This meant that the winner elected would always match up with the “magic best” winner. Since the Bayesian Regret is the difference between the elected candidate and the “magic best”, it was always zero. I hadn’t noticed this earlier because my first method for measuring “unhappiness” returned a non-zero value in every case — there was always somebody unhappy no matter who was elected.
Eventually I found the difference. In Smith’s simulation, even the “honest” voters were using a very simple strategy: giving a max score to the best and a min score to the worst. The reason that the Bayesian Regret for Score Voting is non-zero is due to the scaling of scores between the best and the worst candidates. If a voter strongly supports one candidate and opposes another, then this scaling doesn’t make much of a difference. It does, however, make a big difference when the voters are indifferent between the candidates but gives a large score differential to the candidate that’s slightly better than the rest.
With this observation, it became absolutely clear why Score Voting would minimize Bayesian Regret. The more honest voters are, the closer the Bayesian Regret gets to zero. This raises another question: how much dishonesty can the system tolerate?
Measuring strategic vulnerability
One of the reasons for trying to reproduce this result was to experiment with additional voting strategies outside of the scope of the original study. Wikipedia cites another study by M. Badinski and R. Laraki that suggests Score Voting is more susceptible to tactical voting than alternatives. However, those authors too may be biased towards their proposed method. I think it’s worthwhile to try and replicate that result as well. The issue is that I’m not sure what the appropriate way to measure “strategic vulnerability” would even be.
Measuring the Bayesian Regret of strategic voters and comparing it with honest voters could potentially be a starting point. The problem is how to normalize the difference. With Smith’s own results, the Bayesian Regret of Score Voting increases by 639% by using more complicated voting strategies while Plurality only increases by 188%. The problem with comparing them this way is that the Bayesian Regret of the strategic voters in Score Voting is still lower than the Bayesian Regret of honest Plurality voters. Looking only at the relative increase in Bayesian Regret isn’t a fair comparison.
Is there a better way of measuring “strategic vulnerability”? Bayesian Regret only measure the difference from the “best case scenario”. The very nature of strategic voting is that it shift the result away from the optimal solution. I think that to measure the effects of voting strategy there needs to be some way of taking the “worst case scenario” into consideration also. The normalized regret I discuss above might be a step in the right direction. Any input on this would be appreciated.
Disclaimer
Please don’t take anything said here as gospel. This is a blog post, not a peer-reviewed journal. This is my own personal learning endeavor and I could easily be wrong about many things. I fully accept that and will hopefully learn from those mistakes. If in doubt, experiment independently!
Update: The source code used in this article is available here.